Endogeneity and Gaussian Copulas
Abstract
The Gaussian Copula approach allows researchers and practitioners to detect and correct for endogeneity in PLS-SEM (i.e., for relationships in the structural model).
Brief Description
While endogeneity can have various roots, such as measurement errors, simultaneous causality, common method variance, and (un)observed heterogeneity, endogeneity problems most often arise from omitted variables that correlate with one or more independent variable(s) and the dependent variable(s) in the regression model (Hult et al., 2018). Omitting such variables induces a correlation between the corresponding independent variables and the dependent variables' error term. That is, the independent variables then explain not only the dependent variable, but also the error in the model. In this context, we refer to the terms endogenous and exogenous to identify variables that endogeneity does (not) impact; we use dependent and independent to identify constructs that explain other constructs in partial least squares structural equation modeling (PLS-SEM), or are explained by them.
A simple way to address, or at least reduce, endogeneity is to specify a set of control variables that account for some of the variance in the dependent variable (Ebbes, Papies, & van Heerde, 2017). Despite the careful selection of control variables, researchers must also apply a statistical approach to address endogeneity when a potential endogeneity problem exists. Two broad statistical approaches have been developed to examine the presence of endogeneity: the instrumental variable approach and the instrumental variable-free approach (Papies, Ebbes, & van Heerde, 2017). While the instrumental variable approach has various drawbacks, the instrumental variable-free approaches offer several advantageous features (e.g., Hult et al., 2018). Among the instrumental variable-free approaches, the Gaussian copula method is particularly popular (Becker, Proksch, & Ringle, 2022; Liengaard et al., 2025; Park & Gupta, 2012).
There are two variants of the Gaussian copula approach. The original approach suggests the regression model's estimation by using an adapted maximum likelihood function that accounts for the correlation between the regressor and the error term using the Gaussian copula. The disadvantage of the maximum likelihood approach is that it can only account for one endogenous regressor in the model. In practice, therefore, almost all applications use the second variant, which adds a "copula term" to the regression equation - like the control function approach for IV model estimation. This version has been implemented in SmartPLS. The Gaussian copula control function approach can also account for multiple endogenous regressors, which requires the simultaneous inclusion of multiple copula terms, one for each regressor. The parameter estimate of this copula term is the estimated correlation between the regressor and the error term scaled by the variance of the error. Based on bootstrapped standard errors, a statistical test of this parameter estimate allows for an assessment of whether this correlation is statistically significant and therefore whether endogeneity problems exist (Hult et al., 2018; Papies, Ebbes, & van Heerde, 2017). A key requirement for the application of the Gaussian copula approach is the non-normality of the endogenous variable(s) (Park & Gupta, 2012), which must be checked by researchers and partitions (e.g., via the Cramer-von-Mises non-normality test); see also Becker et al. (2022). Also, in applications, the Gaussian copula has several additional limitations that require careful attention; for details, see Becker et al. (2022); Eckert and Hohberger (2022).
The new and extended framework for the Gaussian copula approach to dealing with endogeneity issues in regression models by Liengaard et al. (2025) addresses several problems and limitations. This approach is implemented in SmartPLS. It can be used not only to address endogeneity issues in regression models, but also for other methods provided by SmartPLS, such as PLS-SEM and path analysis.
Gaussian Copula Approach in SmartPLS
Create or open a PLS path model in SmartPLS. Click the Gaussian Copula button on the menu bar. The click icon appears on each selected relationship in the structural model (see screenshot below). Now, select the relationships in the structural model for which you want to detect and correct endogeneity problems using the Gaussian Copula approach. Left-click on the selected relationship. As a result, a circle labeled GC appears in the model, representing the additional Gaussian copula term for a relationship. Finally, use the PLS-SEM algorithm to estimate the model with the Gaussian copula terms and determine their significance using bootstrapping. Use these results to assess whether there are critical endogeneity problems in the model that are corrected by the Gaussian copula terms.
Note: Note: It is important to check the requirements of the Gaussian copula approach (e.g. non-normality of the endogenous variables) very carefully in each case (Becker et al., 2022; Liengaard et al., 2025).
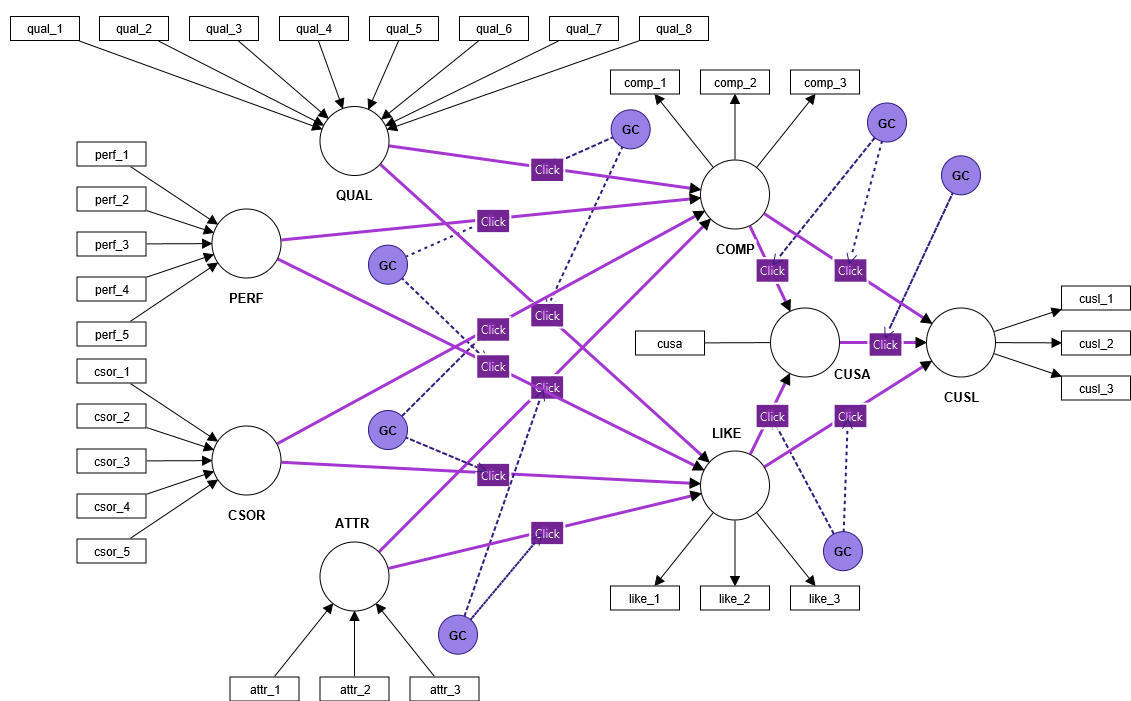
For SmartPLS, we provide sample projects for running the Gaussian copula approach in regression models. Simply download, import, and run these examples in SmartPLS.
References
- Becker, J.-M., Proksch, D., and Ringle, C. M. (2022). Revisiting Gaussian Copulas to Handle Endogenous Regressors. Journal of the Academy of Marketing Science, 50: 46-66.
- Ebbes, P., Papies, D., and van Heerde, H. J. (2017). Dealing with Endogeneity: A Nontechnical Guide for Marketing Researchers. In Handbook of Market Research, C. Homburg, M. Klarmann, and A. Vomberg (eds.), Handbook of Market Research, Springer: Cham.
- Eckert, C., and Hohberger, J. (2022). Addressing Endogeneity Without Instrumental Variables: An Evaluation of the Gaussian Copula Approach for Management Research. Journal of Management, 01492063221085913.
- Hult, G. T. M., Hair, J. F., Proksch, D., Sarstedt, M., Pinkwart, A., and Ringle, C. M. (2018). Addressing Endogeneity in International Marketing Applications of Partial Least Squares Structural Equation Modeling. Journal of International Marketing, 26(3): 1-21.
- Liengaard, B. D., Becker, J.-M., Bennedsen, M., Heiler, P., Taylor, L. N., and Ringle, C. M. (2025). Dealing with Regression Models’ Endogeneity by Means of an Adjusted Estimator for the Gaussian Copula Approach. Journal of the Academy of Marketing Science, 53: 279–299.
- Papies, D., Ebbes, P., and van Heerde, H. J. (2017). Addressing Endogeneity in Marketing Models. In Advanced Methods in Modeling Markets, P. S. H. Leeflang, J. E. Wieringa, T. H. A. Bijmolt, and K. H. Pauwels (eds.), Springer: Cham, pp. 581-627.
- Park, S., & Gupta, S. (2012). Handling Endogenous Regressors by Joint Estimation Using Copulas. Marketing Science, 31(4): 567-586.
- More literature ...
Cite correctly
Please always cite the use of SmartPLS!
Ringle, Christian M., Wende, Sven, & Becker, Jan-Michael. (2024). SmartPLS 4. Bönningstedt: SmartPLS. Retrieved from https://www.smartpls.com